In recent years, the integration of artificial intelligence (AI) in scientific research has brought about unprecedented advancements.
However, as AI becomes an indispensable tool in various scientific disciplines, concerns about its impact on reproducibility have surfaced.
This article delves into the intricate relationship between AI and the reproducibility crisis in science, exploring both challenges and opportunities that arise from this technological integration.
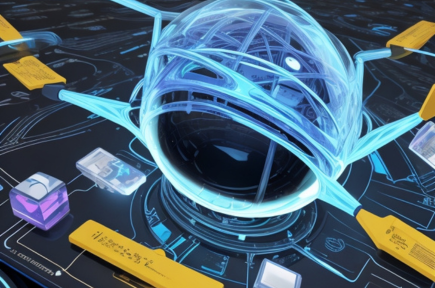
The Rise of AI in Scientific Research
AI has revolutionized the scientific landscape, offering powerful tools for data analysis, pattern recognition, and complex simulations.
Researchers across diverse fields, from medicine to physics, have embraced AI to expedite discoveries and gain insights that were once unattainable.
While these advancements have propelled scientific progress, they also raise questions about the reproducibility of AI-driven studies.
Challenges to Reproducibility in AI-Driven Research
a. Lack of Transparency and Interpretability
One of the primary challenges in AI-driven research is the inherent opacity of certain algorithms.
Many advanced machine learning models operate as ‘black boxes,’ making it difficult for researchers to understand the decision-making process.
This lack of transparency hinders the reproducibility of studies, as other scientists may struggle to replicate and validate the findings.
b. Dataset Bias and Generalization Issues
AI models heavily depend on the datasets used for training. If these datasets contain biases or are not representative of the real-world scenario, the AI system may produce results that lack generalizability.
Reproducing such studies becomes challenging, as the same biases may be perpetuated in subsequent research, leading to a crisis of reliability.
c. Rapid Technological Evolution
The rapid evolution of AI technologies poses another obstacle to reproducibility. The swift development of new algorithms and techniques may render older models obsolete, making it difficult for researchers to replicate studies that employed outdated AI methodologies. This dynamic nature of AI introduces a level of instability in scientific reproducibility.
Opportunities for Enhancing Reproducibility in AI-Driven Research
a. Open-Source Collaboration and Code Sharing
To address issues of transparency, researchers can promote open-source collaboration and code sharing.
Making AI algorithms and models publicly available enhances the reproducibility of studies, allowing other scientists to scrutinize, validate, and build upon existing work.
b. Standardization and Best Practices
Establishing standardized protocols and best practices for AI-driven research can mitigate reproducibility challenges. This includes guidelines for dataset collection, model training, and result interpretation.
By adhering to common standards, researchers can enhance the reliability and replicability of their studies.
c. Robust Validation and Benchmarking
Prioritizing robust validation and benchmarking processes is crucial in ensuring the reproducibility of AI-driven research.
Independent validation of results using different datasets and methodologies can help identify potential biases and improve the generalizability of findings.
Conclusion
As AI continues to shape the future of scientific research, acknowledging and addressing the challenges it poses to reproducibility is imperative.
By fostering a culture of transparency, collaboration, and adherence to best practices, researchers can harness the full potential of AI while maintaining the integrity and reliability of their work.
The ongoing dialogue between the scientific community, policymakers, and technology developers will play a pivotal role in navigating the evolving landscape of AI-driven research and mitigating the reproducibility crisis.
Disclaimer: This article is for informational purposes only. The content provided is based on general knowledge, and readers are advised to seek professional advice for specific situations. The author and publisher do not assume any responsibility for the accuracy or completeness of the information presented. Any reliance on the information provided in this article is at the reader’s own risk.